Scientific projects at the PUEB
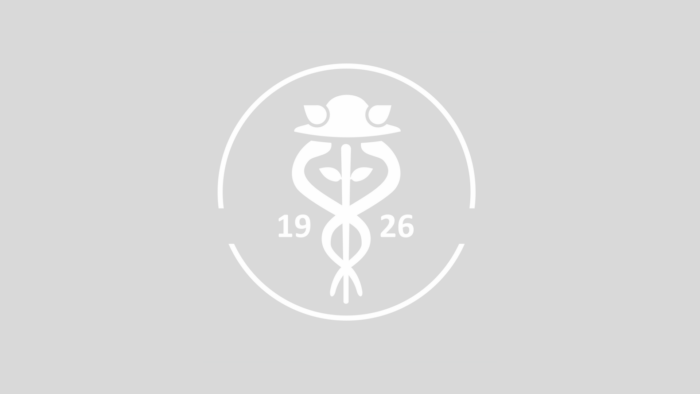
Modelling and Forecasting of Housing Prices
With the growth of the market economy in Poland, some markets which played a marginal role in the pre-transition economic system have grown in importance. The housing market is undoubtedly one of them. The significant role this segment of the real estate market plays in the economy may be attributed to the fact that real estate is perceived as capital, which makes it possible to create added value for the owner as well as for the local and national economy. Moreover, the global financial crisis caused by a collapse in the real estate market in the United States, led to the growing interest in the relationship between the housing market and the economic situation of individual countries. The fact that economists are paying increasing attention to the issue of changes in the real estate market is the result of fears concerning the consequences of the drop in housing prices. The housing market is affected by changes in the economy. On the other hand, through different channels, it can also influence the economic development of regions or countries. Changes in this market affect business cycles, have a significant impact on the transmission of monetary policy impulses, and, under some circumstances, on the stability of the financial system. Studies conducted in various countries show that there is a close connection between fluctuations in this market and changes in business activity in particular countries. Generally speaking, changes in housing prices precede changes in business cycles. Moreover, prices of flats may influence household spending – higher prices make property owners richer, which may, in turn, lead to increased consumption. What is a direct effect of the fluctuations of housing prices in the economic sphere are changes in the volume of real estate investments. An increase in housing prices makes such investments more profitable. Taking into account above-mentioned issues the main objective of the project is to identify and classify the determinants of variation in housing prices in 18 cities (provincial cities) in Poland in years 2000-2017, and on this basis to create price prediction models.
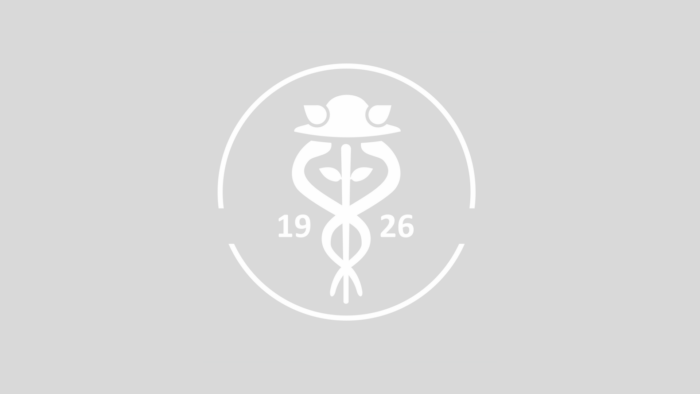
The Quality of Decisions in the Innovation Process in Biotechnological Enterprises
Project Description:
The project “The Quality of Decisions in the Innovation Process in Biotechnological Enterprises” aims to develop a model and comprehensive approach that specifies and contextualizes the innovation process from a decision-making perspective. The primary objective of this approach is to enhance the efficiency of innovation processes in the biotechnology industry, facilitating the regular creation of new products. The lack of a comprehensive theoretical framework hinders the effective development and implementation of sustainable innovation processes, making research in this area both theoretically and practically significant.
This project innovatively integrates research within decision theory. It builds on current discussions around innovation processes and addresses existing research gaps in areas such as:
- Shared interpretative frameworks among innovation process participants,
- Rationality of decision-makers,
- Participation in decision-making processes, and
- Transactional memory.
Biotechnology is widely regarded as a cornerstone of the knowledge-based economy.
The primary role of managers is to make decisions, and the quality of decisions concerning innovation is critical to the effectiveness of the innovation process. In the conceptual model of the innovation process, successive stages are separated by decision points, where managers decide whether to proceed to the next phase. The absence of comprehensive theoretical foundations in this area complicates the effective development of innovation processes. Meanwhile, numerous studies of enterprises confirm that nearly all businesses strive to increase innovation and create organizational solutions leading to the establishment of a continuous innovation process. This process is complex, with each stage involving different approaches to decision-making. Decision-making within the innovation process represents the most significant challenge for companies in the biotechnology sector.
The research problem of the project is expressed as the following question: How does improving the quality of decisions affect the efficiency of the innovation process in biotechnological enterprises? The main objective of the project is to determine how improving the quality of decisions impacts the efficiency of the innovation process in biotechnological companies. This objective is supplemented by ten specific goals. The main hypothesis states: The quality of innovation-related decisions impacts the efficiency of the innovation process in such a way that higher decision quality contributes to increased process efficiency.
The research plan proposed in the project is divided into seven parts, ultimately creating a comprehensive view of the innovation process from the perspective of decision quality in biotechnological enterprises.
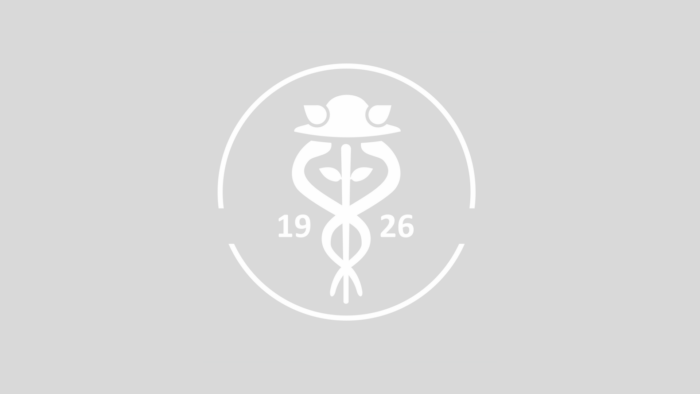
The influence of digitalisation of the economy on skills, competences, and relationships at the workplace
Project Description:
The main goal of the project is to determine the role of EU authorities, national governments, and social dialogue in the process of digitalizing the global economy. This project aims to develop recommendations and best practices that can be disseminated at the European level.
The project is based on research and analyses from four perspectives: European, business, employees, job seekers, and trade unions.
The research framework includes the following key questions:
- What challenges do stakeholders face in the process of employees acquiring new skills?
- Are there national public policies (education and training) agreed upon with social partners that aim to improve the efficiency of acquiring the skills and qualifications required for new jobs created by digitalization?
- Does digitalization affect the quality of employment?
- Does it create new forms of employment, and does it lead to instability and job insecurity?
- Does digitalization contribute to increased risks and income inequality, causing lower wages for less-educated workers?
- What is the role of collective interest representation in adapting to technological changes and their impact on employment and labor relations?
- Does digitalization create barriers for employees to access collective interest representation?
- The obtained results will allow for a comprehensive characterization of labor relations and the activities of authorities in coordinating digitalization processes. They will also indicate potential ways to measure and stimulate this process in EU countries by leveraging the synergy and diversity of the consortium’s work.
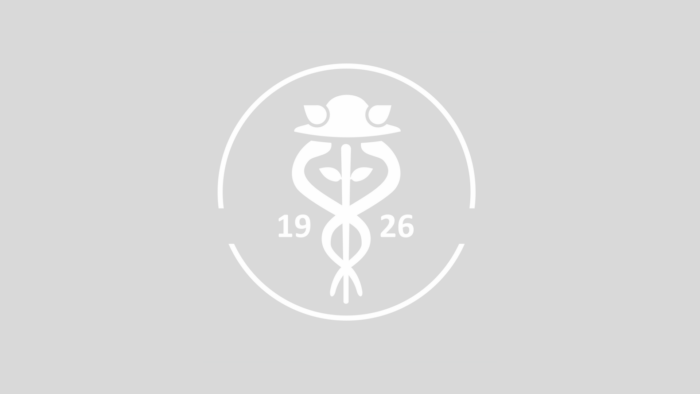
The Impact of Support Received by Organic Farms under the “Organic Farming” Programme
Project Description:
The aim of the project is to determine the impact of support received by organic farms under the “Organic Farming” program, part of the Rural Development Program (RDP) for 2014-2020, on the changes in production levels in organic farms in selected provinces (Greater Poland and Lublin Voivodeships). Additionally, it seeks to identify how this support influences changes in the supply of organic food in Poland. Furthermore, the research will allow for the determination of:
- the potential for further increasing the supply of organic food through the utilization of funds allocated under the RDP;
- whether consumers perceive changes in the availability of various categories of organic food products.
Quantitative and qualitative research will be conducted among representatives of the supply side (farmers, processors, distributors) and the demand side (consumers) of the organic food market, as well as among experts.
The results of the supported project will be made freely available to all enterprises operating in the organic farming sector or related agricultural or forestry sub-sectors on the project’s website.
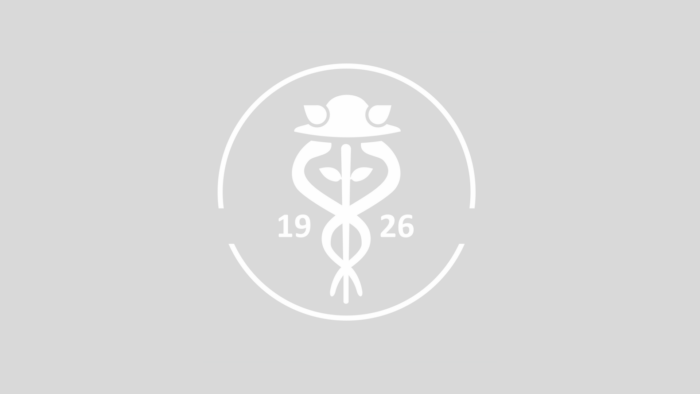
The properties of risk premia in the commodity market
The study aims to analyze a broad range of return patterns documented previously in the other asset classes and to verify whether they also apply to the commodity market. The examined patterns would form an investment universe for further studies on their properties, including their predictability with various variables, the impact of trading costs and illiquidity on their performance, explanatory power over them of well-known factor pricing models, a contribution of various phenomena to the development of the return patterns. The research is going to focus on the newest developments from the past 10-15 years in the area of asset pricing on the financial markets. The main hypothesis of this study is that: The cross-sectional variation in commodity returns can be explained with risk factors, which have their documented parallels in other asset classes.
The main aim is going to be achieved through the analysis of the various fragmentary research hypotheses. The detailed studies will include 1) investigation of momentum and low-risk effects in the commodity risk factor returns 2) examination of the influence of the time-series variation in the marketwide investor sentiment and limits on arbitrage on the factor performance in the commodity market, 3) investigation of seasonal effects in cross-sectional asset pricing factors in the commodity market, 4) investigation of momentum strategy based on return residuals derived from asset pricing models (alpha momentum) on commodity market, 5) examination of the long-term reversal effect occurrence in commodities returns on above seven centuries long dataset , 6) investigation of inflation hedging effect influence commodities returns on above seven centuries long dataset.
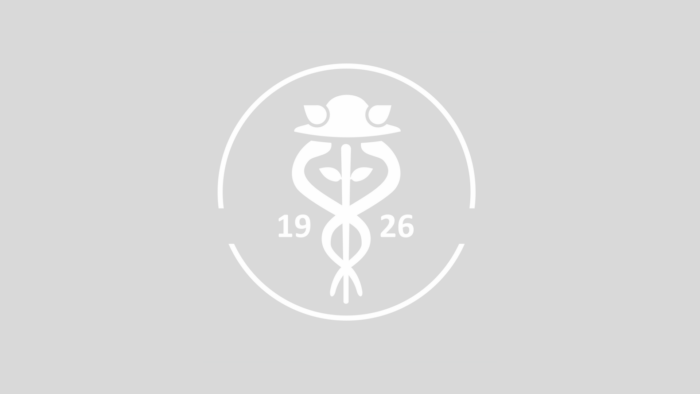
School-to-work transition in a comparative perspective
SCHOOL-TO-WORK TRANSITION IN A COMPARATIVE PERSPECTIVE
School-to-work transition (SWT) can be defined as a period lasting from few months to few years between graduating from formal education and the beginning of stable employment. This period is characterized by high labour market and employment mobility. During the SWT phase we frequently change jobs, lose jobs, get promotions. We also take important life decisions, about leaving the family house, moving abroad, starting own family. The smoothness of the SWT influences our entire life. We can easily imagine that the problems with finding a decent job at the beginning of the professional career will have negative consequences for our entire professional life. From this reason scientists carefully investigate the conditions of SWT since the knowledge how to improve the labour market entry process will help to avoid future problems. In this research we deal with few aspects of SWT. Firstly, we try to design new measures of SWT allowing to capture its dynamics and complexity. Secondly, we focus on some consequences of early job insecurity, e.g. how the problems with employment influence transitions into parenthood or how the employers, reading job applications, perceive the frequent job changes of candidates. Finally, we try to understand what models of SWT transition represent post-socialist countries.
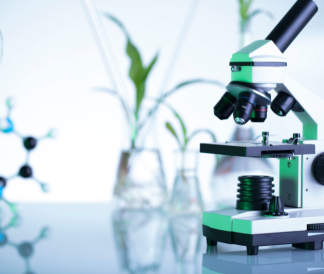
Development of novel derivatives of otilonium bromide as antifungal agents
Project Leader: dr hab. inż. Daria Wieczorek, prof. UEP
Project Description:
The search for new substances with biocidal activity is one of the most significant challenges in global science, especially due to the growing resistance of microorganisms to existing biocides. The aim of this project is to develop new antifungal agents based on modifications of otilonium bromide (OB), to elucidate the mechanisms of antifungal activity, and to determine the cytotoxicity and therapeutic efficacy of OB derivatives.
Otilonium bromide is an antispasmodic compound from the family of quaternary ammonium derivatives and is successfully used in the treatment of patients with irritable bowel syndrome. However, our team was the first to discover that OB exhibits anticryptococcal activity and demonstrates other antifungal properties. This discovery provides a solid foundation for further research into the antifungal activity of OB and its derivatives. The project plans to synthesize OB derivatives, determine their mechanism of action and antifungal properties, and subsequently assess their cytotoxicity and therapeutic efficacy. The results obtained will enable the characterization of antifungal activity across a broad group of OB derivatives and highlight their potential as therapeutic agents.
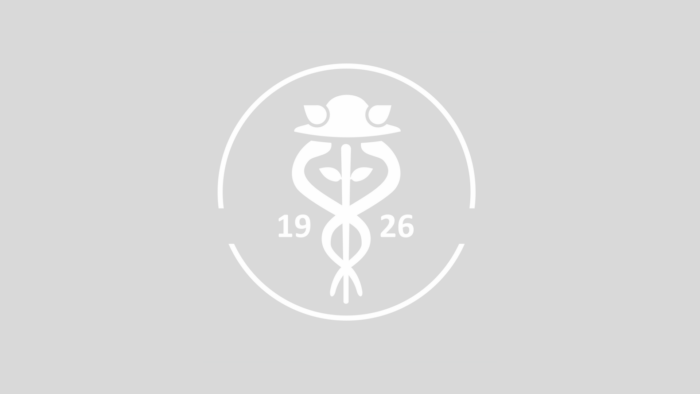
Institutional investor monitoring and corporate financial decisions in CEE countries
Project team leader: dr hab. Tomasz Jewartowski, prof. UEP
Public companies from CEE countries have much more concentrated ownership and are typically controlled by founding families or states. Equity stakes held by institutional investors are much lower in CEE countries than in developed markets. Moreover, there are substantial differences among countries (e.g., institutional investors hold approximately 33% of public equity in Poland and only 1% in the Slovak Republic). The major corporate governance conflict is between the controlling shareholder and minority shareholders. Institutional investors are thus supposed to monitor the managers appointed by controlling shareholders and discourage them from expropriating minority shareholders. Nevertheless, institutional investors are not homogeneous, differing in regard to investment horizons and policies, size, location, and portfolio concentration – all of which lead to different attitudes and abilities to serve as monitors.
In our project, we would like to answer the following questions: What factors influence the ownership decision of institutional investors in Central and Eastern Europe (CEE)? How do different types of institutional investors affect corporate policies in CEE countries? How do institutional investors exert their influence on public companies listed on CEE stock exchanges?
We expect that the effectiveness of institutional investors’ monitoring via traditional ‘voice’ or ‘exit’ channels are on average relatively weak because of lower shareholder protections, legal enforcement, and market liquidity in CEE countries as a consequence of ubiquitous control enhancing mechanisms such as pyramid structures and dual-class shares. Also, we expect to confirm that institutional investors can exert influence on companies in CEE countries using an alternative channel – capital structure. Institutional investors can influence companies because they typically have good relationships with banks, who are the main providers of debt in CEE countries due to underdeveloped bond markets. Moreover, institutional investors can share ownership of
companies with banks.
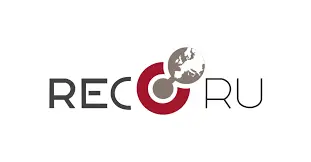
Regional policy in Europe in conditions of development shocks – challenges of regional development resulting from the socio-economic consequences of Russia’s aggression against Ukraine
Project Team Leader: Prof. dr hab. Paweł Churski UAM Research Team: https://recoru.web.amu.edu.pl/zespol-badawczy/
Contemporary development shocks are definitely deeper than those observed in the 20th century, mainly due to the much higher degree of interdependence of the modern world and the presence of global challenges related to climate change in the era of globalization. The consequences of economic crises and development shocks are spatially diverse, affecting regions with a lower potential or relatively stronger level of development. Therefore, they may accelerate the degree of socio-economic divergence, which creates new challenges for the cohesion policy in the European Union and for the processes of building/strengthening the long-term resilience of individual EU regions. The main objective of the project is to identify spatial differences in selected social and economic consequences of Russia’s aggression against Ukraine and to explain their impact on the transformation of the regional development process and the evolution of regional policy in the European Union’s (EU) countries and regions. The main objective has been divided into specific cognitive, methodological and application objectives.
The research will be carried out in 3 spatial arrangements: EU Member States, regions corresponding to the NUTS2 level and selected test regions in 2 countries bordering Ukraine (Poland, Finland, Romania), and 1 EU core country (Germany). The time range of the analysis will cover the years 2014-2023, i.e. the period of 3 main shocks: Russia’s aggression against Ukraine, SARS-Co-V2, and the financial crisis.
Research Team: https://recoru.web.amu.edu.pl/zespol-badawczy/
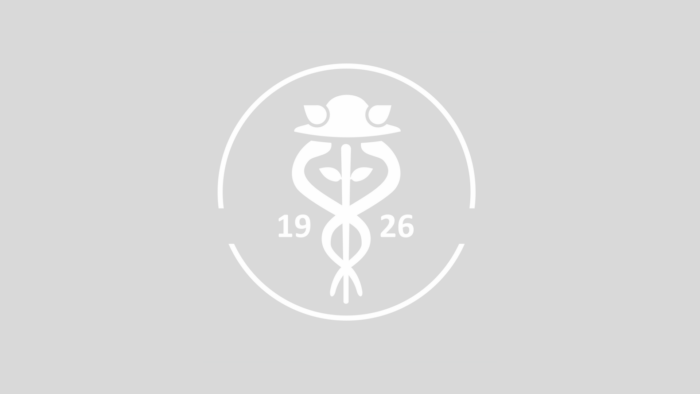
The trajectories and challenges of the development of inner peripheries in the new conditions of cohesion post SARS-CoV-2
Domestic and regional differences in the level and dynamics of development have been subject to extensive
research within economics and economic geography. The SARS-CoV-2 pandemic will significantly impact
these differences, leading to multi-faceted economic and social consequences. The negative consequences to
the so-called “inner peripheries” (permanently marginalised areas) may be particularly important. These areas
find it difficult to follow the growing economic cores located around big cities. The recent pandemic may
accelerate the social and economic divergence between these areas which will be undesirable from the point
of view of sustainable development.
The aim of the project is to identify the regularities of changes to the socio-economic development in selected
inner peripheries in Poland with special attention given to their sensitivity and resilience to the consequences
of the SARS-CoV-2 crisis, and the resulting recommendations for the changes in the development policy
interventions. The main goal of the project has been broken down to specific objectives of cognitive,
methodological and application-related nature.
In the project, a novel methodological approach has been adopted. Research team will use yet unpublished
data from the Ministry of Finance, data from the System of Self-Governmental Analyses and the results of in-
depth analyses provided by the Association of Polish Cities. In combination with an original geospatial
database of open and public data, and data provided by the Central Statistical Office on multi-faceted spatial
access to services and jobs, it should lead to a better understanding of the transmission of the effects of the
SARS-CoV-2 crisis on the specific industries and sectors of the economy as well as the inhabitants’ income.
Among the applied analytical approaches, also an innovative multi-variant approach to systematising inner
peripheries in Poland will be employed. It will take into consideration the impact of functional regions of
medium-sized and small towns on the surrounding underdeveloped areas. By making use of generally
available and unpublished sources of quantitative data it will be possible to apply models of spatial regression,
previously not used to this end or to this extent on a local level. Another value of the planned research is the
adopted structure based on identifying the factors of peripherality (factual and potential), and creating a system
monitoring the multi-faceted changes to inner peripheries in the country.